Every hour, Walmart processes 2.5 petabytes of customer data from 245 million shoppers worldwide. The challenge and risks were massive: managing and storing such massive data volumes required efficient infrastructure, unifying unstructured data from various touchpoints was complex, and delays in insights led to lost opportunities and inefficient inventory management. But then, Walmart tackled it by creating the world's largest private cloud, called Data Café, at their Arkansas headquarters.
This system analyzes over 200 data streams, scanning through 200 billion rows of transactional data in microseconds. As a result, Walmart saw a 10-15% increase in online sales and $1 billion in additional revenue. If you doubt the power of predictive analytics in retail, this story illustrates it well.
In 2025, behavior prediction is a cornerstone of effective retail strategy. Let’s dig into predictive retail, its methodologies, and its connection to AI. We will also walk through the fundamental steps of implementing predictive systems in retail.
How does predictive analytics work?
The foundation of retail predictive analytics rests on four pillars: data collection, cleaning, modeling, and interpretation. Let's see how each stage transforms raw information into actionable insights.
- Data collection
Modern retail generates a massive volume of data across multiple touchpoints. Every transaction, website click, inventory movement, and interaction is a data point. Physical stores collect data through point-of-sale systems, security cameras, and foot traffic sensors. Online platforms track browsing patterns, cart abandonments, and purchase histories. Social media interactions and customer service conversations contribute to this vast data ecosystem.
- Data cleaning
Raw data rarely arrives in a usable format. The cleaning phase transforms it into a structured dataset, cutting duplicates and standardizing formats. When running customer behavior analysis, the system must recognize that "NYC," "New York City," and "Manhattan" refer to the same location. Similarly, product descriptions need standardization – "women's blue sweater M" and "medium ladies pullover navy" are the same item.
- Modeling
Once cleaned, the data feeds into sophisticated modeling systems that uncover patterns and relationships. Predictive behavior modeling employs various techniques:
- Statistical analysis examines historical sales patterns to forecast future demand.
- Machine learning algorithms identify complex patterns in customer behavior.
- Neural networks process massive datasets to recognize subtle correlations.
- Time series analysis tracks seasonal trends and cyclical patterns in retail performance.
A model might combine seasonal purchasing patterns with local weather forecasts and economic indicators to predict demand for specific product categories.
- Interpretation
Here, sophisticated visualization tools and reporting systems present findings in accessible formats. Retail managers receive clear recommendations rather than raw statistical data. For instance, instead of showing complex algorithms, the system might recommend: "Increase inventory of winter boots by 25% in Northeast stores within the next two weeks based on predicted early snowfall."
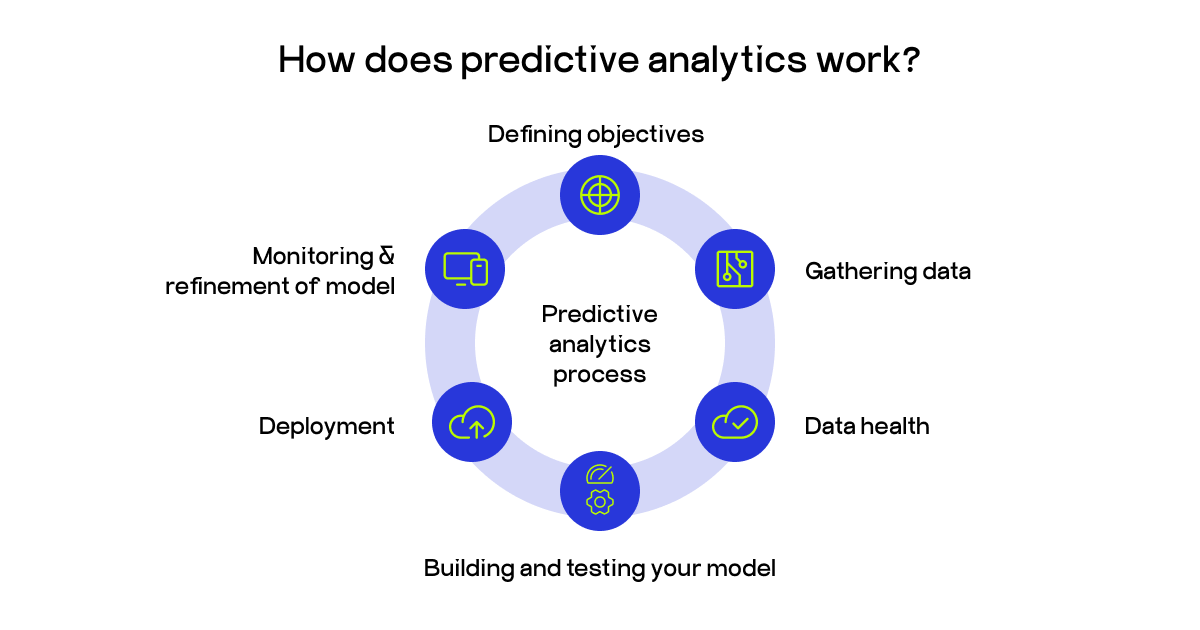
This interpretation phase also includes continuous feedback loops. As predictions meet reality, the system learns and adjusts. When a forecast proves accurate, correctly predicting a surge in umbrella sales before an unexpected rainy season, the model strengthens predictive pathways. On the flip side, missed predictions trigger analysis and refinement of the given assumptions.
4 types of retail analytics
Retail analytics unfolds across four distinctive approaches, each offering unique insights for business growth. Let's explore how these analytical methods revolutionize retail decision-making.
- Descriptive analytics: It illuminates past performance through clear metrics and straightforward reports. This foundational approach answers essential questions about sales patterns, inventory movement, and customer interactions. Weekly dashboards showcase vital statistics, creating a solid base for deeper analysis.
- Diagnostic analytics: When something goes wrong, diagnostic analytics steps in as your retail detective. By weaving together diverse data threads — from customer feedback to financial metrics — it uncovers the hidden stories behind performance issues. This analytical sleuth helps pinpoint exactly why sales dipped in certain regions or why specific products underperformed.
- Predictive analytics: Here's where retail gets fascinating. Predictive analytics is your business fortune teller, as it uses sophisticated data models. It considers countless variables — economic shifts, weather patterns, competitive moves — to forecast scenarios. Want to know how different discount strategies might play out? This is your tool.
- Prescriptive analytics: The crown jewel of retail analytics, prescriptive analytics combines artificial intelligence with big data to transform predictions into action plans. It empowers customer service teams with real-time recommendations and helps managers make decisions about everything from inventory levels to promotional strategies.

Each analytical approach builds upon the others, creating a comprehensive toolkit for modern retailers. And this toolkit is vast, covering the most diverse applications.
Core methodologies driving retail intelligence
In the world of predictive analytics for retail, three major methodologies form the basics of modern retail intelligence systems. Each plays a unique role in transforming raw data into actionable insights.
- Probabilistic modeling calculates the likelihood of future customer actions by analyzing past behaviors. For instance, when conducting retail store analysis, models predict that a customer who purchases baby clothes is likely to buy the next size up within three months. This insight enables stores to time their promotional offers, reaching customers exactly when they're ready to buy.
- Clustering models work like sophisticated customer sorting systems. Through analytics for the retail industry, these models group shoppers with similar habits, preferences, and behaviors. One group might be bargain hunters who shop exclusively during sales, while another consists of trendsetters who prioritize new arrivals regardless of price.
- How do technological advances influence consumer decisions? This question leads us to collaborative filtering, the most familiar methodology to modern shoppers. It's the technology behind those "you might also like" suggestions. When a customer browses winter boots, the system might suggest warm socks or water-proofing spray, based on what similar customers purchased together.
The predictive analytics retail magic lies in how these methodologies work together. While probabilistic models might identify when a customer is likely to make their next purchase, clustering helps determine what products to recommend, and collaborative filtering suggests the perfect complementary items. This synchronized approach creates a shopping experience that feels personally curated for each customer while helping retailers optimize their inventory and marketing efforts.
AI and Machine Learning in retail predictive analytics
Machine learning and artificial intelligence have changed the very face of predictive analytics in retail stores, as it gives much better ways for businesses to understand and serve their customers.
The brain of the operation, machine learning algorithms, processes vast amounts of historical sales data to spot patterns humans might miss. For instance, these algorithms might notice that customers who buy running shoes typically return for replacement pairs every eight months – information that helps stores time their promotional offers perfectly.
Pro tip: AI works in real time, creating a feedback loop that continuously improves accuracy. We mentioned these loops before — but it’s specifically the machine learning capabilities allowing such iteration and continuous improvement. Implementing specialized AI increases your chances of hitting the right spot with data accuracy and precision.
Natural language processing (NLP) acts as the system's ears and voice, analyzing customer reviews, social media comments, and support interactions. Through AI behavior analysis, it can detect subtle shifts in customer sentiment – perhaps revealing that shoppers are increasingly mentioning "sustainability" in their product reviews, signaling a growing trend.
Serving as the system's eyes, computer vision monitors in-store traffic patterns, shelf inventory levels, and even customer interactions with products. Imagine cameras that can tell when a display is drawing attention but not converting to sales, suggesting a potential pricing or placement issue.
In a nutshell, there are multiple options available, from custom ML models to off-the-shelf LLM integrations, so choose wisely. Consulting AI development and integration experts will work for you, if you want to invest in the 100% right solution that aligns with your expectations. Sometimes, this means investing more at the start to future-proof your infrastructure, which later results in lower maintenance and optimization costs.
